Emily Getzen
Mining for Health: A Comparison of Word Embedding Methods for Electronic Health Records
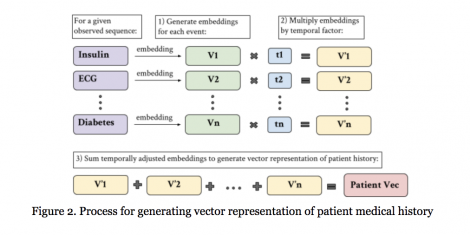
Presenter
Flash Talk Presenter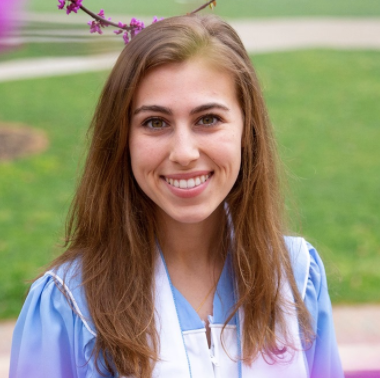
I am currently a second-year Biostatistics PhD student at the University of Pennsylvania under the mentorship of Dr. Qi Long. My research interests primarily lie in the use of statistical and machine learning methods for analysis of electronic health records (EHRs) to advance early-stage disease prediction.
Abstract
Electronic health records (EHRs), routinely collected as part of healthcare delivery, offer great promises for advancing precision health. At the same time, they present significant analytical challenges. EHRs contain multi-scale data from multiple domains. Data for individual patients are collected at irregular time intervals and with varying frequencies. To tackle these challenges, advanced statistical and machine learning methods have been developed to harness the power of EHRs, for example, for predicting diagnoses earlier and more accurately. One powerful tool for extracting useful information from rich, yet complex EHRs data is word embedding algorithms—these algorithms represent words as vectors of real numbers while also capturing and preserving word relationships and semantic and syntactic similarities in the context of EHRs. This can be viewed as automated feature extraction. The resulting features can be used for say predictive modeling of medical events. While currently there exist a wide variety of word embedding tools such as Word2Vec, BERT, FastText, ELMo, and GloVe, there has been little to no work on comparing their performance for analysis of EHRs data. We extend the word embedding methods to embed a patient’s entire medical history, and use the resultant embeddings to build prediction models for medical events. We assess performance of multiple advanced embedding methods in terms of predictive accuracy and computation time using the Medical Information Mart for Intensive Care (MIMIC) database. Our results provide guidance to practitioners regarding the use of word embedding methods for analysis of EHRs data.
Keywords
EHRs; NLP; Word EmbeddingCommenting is now closed.
About Us
To understand health and disease today, we need new thinking and novel science —the kind we create when multiple disciplines work together from the ground up. That is why this department has put forward a bold vision in population-health science: a single academic home for biostatistics, epidemiology and informatics.
© 2023 Trustees of the University of Pennsylvania. All rights reserved.. | Disclaimer