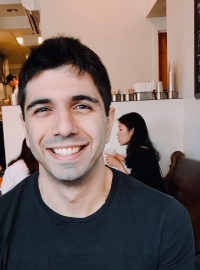
Hometown: Born in Yerevan, Armenia; grew up in Worcester, MA
Education: BA in Quantitative Economics, Providence College
MS in Biostatistics, University of Pennsylvania
Pursuing PhD in Biostatistics
From the 2008 financial crash to biostatistics: After college, I worked for a consulting firm in financial practice, using statistical models to assess the economic risk of derivatives that went toxic during the 2008 crisis. When that work started drying up, I moved to the healthcare practice, where we used statistical models to assess the risk and effectiveness of drugs our pharmaceutical clients developed. (That work wasn’t so different, it turned out. I realized what I cared about most was statistical modeling.)
I developed a real passion for biostatistics—to the point where I was reading textbooks for fun. Most of my managers had PhDs in biostatistics, and they encouraged me to apply to programs.
Why Penn biostatistics: I was interested in causal inference, and I knew Penn had the Center for Causal Inference, a Causal reading group with members from the biostatistics and Wharton statistics faculties, and many pioneers in causal inference. I felt I’d have really good mentors and I do. My advisors, [Professor] Nandita Mitra and [Adjunct Professor] Jason Roy, have been immensely valuable and supportive.
Economics? Statistics? How about both: Economics taught me a principled, methodical framework for thinking about prices and costs. If I think healthcare service prices are “too high,” for example, I can ask myself which assumptions are being violated in the real world that are causing them to deviate from the ideal.
From statistics I’ve learned, especially, the importance of defining a study question precisely. Say we want to estimate the healthcare costs associated with a particular treatment. We should be very precise with what we mean by “costs.” Costs for the insurer? The patient? The provider? Costs accumulated over what follow-up period? What types of costs: inpatient, outpatient, prescriptions? If we don’t understand precisely what we’re measuring, our study is doomed from the beginning.
Why we need a method that doesn’t assume much: Right now my colleagues and I are comparing healthcare costs of different treatments for endometrial cancer, and also investigating hospitalization costs specifically. These are challenging problems. The sticker cost of one treatment may be much higher, but if it’s associated with fewer follow-up hospitalizations, it may be associated with lower total costs overall. Patients assigned to a given treatment may just be sicker than those assigned to another. There can be many patients with little-to-no costs and clusters of very high-cost patients.
Modeling these costs becomes very difficult. If we assumed, for example, that costs have a bell-shaped distribution, we would be very wrong. Bayesian nonparametric methods address this by making very few assumptions about cost distributions. So they can capture the complexities of cost data, yielding better estimates of causal effect—which is what we care about in health policy.