Garam Lee
Artificial Intelligence and Individualized Optimal Glycemic Target in ICU Patients
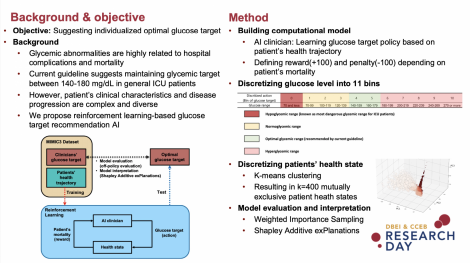
Presenter
Flash Talk Presenter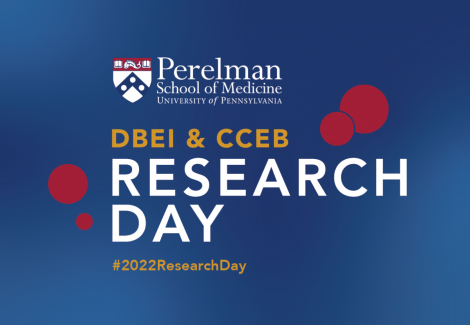
Postdoctoral researcher
Reserch Interest: Time series data analysis, model interpretation
Abstract
Background: The presence of glycemic abnormalities including diabetes, stress-induced hyperglycemia, and hypoglycemia is closely related to increased risk of hospital complications and mortality. Previous studies have demonstrated the deleterious effects of poor glycemic control in critically ill patients. Thus, glycemic control has been considered as a major factor for critical care management.
Current guideline suggests maintaining the glycemic target between 140-180 mg/dL in general ICU patients. However, clinical characteristics and progression patterns of critically ill patients are very diverse and complex. This limits to perform optimal glycemic control relying on the judgement of the clinician. To support clinician’s decision for optimizing glycemic control in critically ill patients we propose reinforcement learning-based model that suggests patient-level optimal glycemic target.
Method: We developed a clinical decision support model using Temporal Difference learning algorithm to learn glycemic target based on observations in existing records. The proposed model was validated using two independent intensive care unit databases: Medical Information for Mart for Intensive Care III (MIMIC-III) and eICU Collaborative Research Databases (eICU-CRD). We compared the performance of our model (AI) to clinician’s glycemic control policy. Performance was measured using Weighted Importance Sampling (WIS) which enables to evaluate consecutive glycemic targets depending on patient’s mortality. Finally, Shapley Additive exPlanations (SHAP) value was used to measure the feature importance for machine’s decision-making.
Result: Our model suggests per-day optimal glycemic target and expected return ranging from -100 to 100 where return value closed to 100 is likely to survive while -100 is likely to be death. Our model estimated expected return based on patient’s mortality. Good model calibration was also confirmed by plotting the relationship between the return of the clinician’s suggestion and mortality. Furthermore, we compared glycemic target distribution recommended from clinician and AI. The most frequent optimal glycemic target range determined by AI was 120-139 mg/dL, which was lower than the recommendation of current guideline. However, the optimal glucose range recommended by AI was varied. For feature importance analysis, Glasgow coma score, age, and platelet count were measured as the most significant factors to determine glycemic target.
Conclusion: Our study proposed reinforcement learning-based glycemic target recommendation model. Results showed that the expected return estimated from AI explains mortality rate well. To interpret AI suggestion, we used to identify the major factors for machine’s decision-making.
Keywords
Reinforcement learning, individualized glycemic control, temporal-difference learningAbout Us
To understand health and disease today, we need new thinking and novel science —the kind we create when multiple disciplines work together from the ground up. That is why this department has put forward a bold vision in population-health science: a single academic home for biostatistics, epidemiology and informatics.
© 2023 Trustees of the University of Pennsylvania. All rights reserved.. | Disclaimer