Siddharth Rawal
Transformer-based Sentence Segmentation for Clinical Notes

Presenter
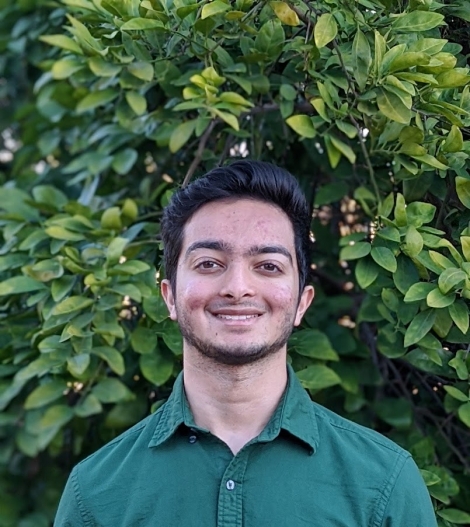
Sid Rawal is a first-year Master's student studying Computer and Information Science at the University of Pennsylvania. He is working under the guidance of Dr. Graciela Gonzalez-Hernandez in the Health Language Processing Lab. His research interests lie in using natural language processing towards applications in healthcare.
Abstract
Sentence segmentation, also known as sentence tokenization or sentence boundary disambiguation, is the task of splitting text into sentences. Sentence segmentation systems rely on rules that are either written by humans or learned automatically from annotated text data to identify occurrences of patterns within text. Since sentence segmentation is typically an early step in natural language processing (NLP) pipelines, performance of a sentence segmentation system can significantly influence performance on downstream tasks. In an NLP pipeline for processing clinical notes, sentence segmentation offers value for various tasks such as identifying discussions of certain topics during patient visits. However, because clinical notes do not follow the same structural and writing conventions as regular English text, approaches for many common NLP tasks in the general English domain do not yield similar performance when applied to clinical notes. Text structures that are common in clinical notes but absent in general English, such as medical abbreviations, numbered lists, and sentences terminated by new lines, can cause general sentence segmentation systems to perform poorly on clinical note corpora. To address the task of sentence segmentation in the clinical note domain, we introduce a transformer-based sentence segmentation model trained on notes from the MIMIC-III clinical database and compare its performance to those of existing systems. Evaluation is currently under way. We will evaluate the systems by collecting intrinsic metrics (precision, recall, and F1-score) and will also perform an extrinsic evaluation by exploring the use of sentence segmentation for identifying mentions of goal-of-care discussions within clinical notes.
Keywords
natural language processing, sentence segmentation, clinical notes, machine learning, deep learningAbout Us
To understand health and disease today, we need new thinking and novel science —the kind we create when multiple disciplines work together from the ground up. That is why this department has put forward a bold vision in population-health science: a single academic home for biostatistics, epidemiology and informatics.
© 2023 Trustees of the University of Pennsylvania. All rights reserved.. | Disclaimer